In the previous article we looked at how to get started with SharePoint Syntex, covering in particular the initial steps of creating a document understanding model. In this article we'll look at how Syntex can extract content from your documents - allowing you to unlock "golden" information so people don't have to open 10 documents to find what they're looking for. Before we get into things, remember that a document understanding model can have two elements:
- A classifier - this allows Syntex to identify what type of document it is (e.g. a "C+C Statement of Work" in the example I'm using)
- An entity extractor - unsurprisingly, this allows Syntex to extract information once trained
We'll focus on the entity extractor today, and this is the fun part. If you remember our scenario from the last article, I'm extracting the total value from each Statement of Work document I have in Office 365. Here's what that looks like - it's the 3rd highlighted rectangle here:
If you remember, creating both a classifier and an extractor uses this process:
Syntex needs some training files to use as we're developing the AI model, but in my case I added these last time when I created the model initially and defined the classifier. As you might imagine, these are some test Statement of Work documents with one or two others thrown in there - the "others" are used to train Syntex about "negative" cases. These go into a special "Training Files" library within the Content Center, and I'll use those same files for the extractor.
Implementing an entity extractor in the AI model
The first step is to head back to the Content Center and find the model you're adding the extractor to:
Once in the model, choose the "Create and train extractors" action:
Next, name your extractor and specify if you want the data to be extracted to a new column on the SharePoint library (and the data type if so) - usually you do. Since I'm extracting the total value from each Statement of Work, so the name I use is "Engagement value":
We're then taken into the "Label" tab, the first step of three when defining a classifier or extractor.
Creating the extractor - labelling step
Accuracy requires labelling and "explanations"
When labelling your files for an extractor, you are teaching Syntex where the value is in your sample files. But as we'll see, simply showing Syntex where it is in a couple of files isn't enough. We need to create "explanations" too - the AI engine uses both pieces of info.
Here, we are dealing with the labelling step.
In the labelling tool (where all formatting is removed from the document), I find the costs table which is present in all of our Statements of Work and I highlight the value from the total row:
I then hit the "Next file" button and repeat for the next document in the training files library:
Once I've labelled at least five files, I move to the "Train" tab.
Creating the extractor - explanations step
For the training part of the process, we create one or more explanations to help guide the AI further. When we created explanations for the classifier, we were providing Syntex with patterns to help identify and classify the document. For the extractor, we do something similar but here we are providing patterns to guide Syntex to the content we are trying to extract.
Explanations can be created from scratch or from a template:
Templates already exist in the system for common pieces of info you may want to pull out of documents - for example, dates, numbers, phone numbers, addresses and so on:
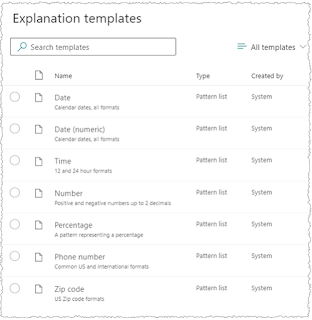
For the sake of learning I'll create my explanations from scratch, even though the first one is actually a currency value and a template exists for that. I give it a name, choose the Pattern list type and provide the variants to account for how the engagement value may be written in my documents (different number formats):
I then save this explanation and create another one. This time I'm helping the AI find the overall section within our SOW documents which the costs table can be found in - I'm simply looking for the title of that section, "Fees and Payment":
I create one more to find the phrase "Total".
Now that I have all of those, I combine them so that I can essentially say "first, please find the phrase 'Fees and Payment', then 'Total', THEN the thing that looks like a GBP currency value. I do this by creating a new explanation of type "Proximity" - and specifying how far apart each element is. Syntex uses the concept of
tokens to specify proximity, and my resulting explanation looks like this:
More accurately, I'm saying "first find the 'Fees and Payment' phrase, then find 'Total' which is more than 20 tokens away but less than 100. Once there, find the thing that looks like a GBP currency value which is VERY close, in fact less than 10 tokens away.
As you can imagine, tuning the tokens in a proximity explanation helps the accuracy of the AI and reduces the chances of Syntex being unable to find your content. My final set of explanations looks like this - it's the 3 phrase or pattern explanations AND the proximity explanation which combines the others:
Creating the extractor - training/testing step
I'm now ready to train and test. Similar to when I did this for the classifier, I select some training files which haven't been used in labelling (including one document that isn't a Statement of Work):
The "Prediction" column then tells me what Syntex predicts would be the extracted text for each document. Success! This looks good:
That's almost a 100% success rate - but you might notice that the model failed to extract content from one SOW document, and indeed Syntex tells me this:
Upon further inspection, this particular document seems to have a structure different to what I'm expecting - specifically, I find that the author has used a different heading for this section of the document!
So at least I understand why this is happening - I can now tweak my explanations if this is an expected case, or politely remind the project manager that they should be following our standard structure! Either way, there's a path to resolving this.
I now finish the process by clicking on the "Exit training" button:
Seeing results - applying the model to document libraries
Our work is now done! We have a completed AI model and we can apply it to document libraries around the Microsoft 365 tenant:
A Syntex AI model does need to be applied to libraries individually, but in most cases your documents of a certain type may not be distributed that widely anyway. In the future, we can expect APIs and provisioning mechanisms to manage this at scale.
Once the model has been applied, Syntex extracts the content I trained it to - meaning I don't need to open each individual document:
Summary
We've now seen the process of creating a document understanding model in SharePoint Syntex - something that will allow us to recognise the document AND extract content from it. We can take this further too. Instead of just extracting a single piece of information (e.g. the value from a Statement of Work) we can, of course, extract
multiple pieces in the same extractor.
Overall, these capabilities of Syntex provide a great leap forward in terms of how information can be found. High value information no longer needs to be buried inside documents, meaning that employees either do not see it or are forced to open many individual documents to find it. We can create mini-databases and tools from content that was previously locked away - including capabilities which provide sorting, filtering and powerful search experiences. To the future!