We’re all familiar with increasing rates of data growth, and
practically every organization using Microsoft 365 has an ever-growing tenant
which is accumulating Teams, sites, documents and other files by the day. The
majority of organizations aren’t making the most of this data though – in the
worst cases it could just be another digital landfill, but even in the best
case the content grows but doesn’t have much around it in terms of intelligent
processing or content services. As a result, huge swathes of content go
unnoticed, search gives a poor experience and employees struggle to find what
they’re looking for. This is significant given that McKinsey believe the average knowledge worker spends nearly 20% of their time looking for internal information or tracking down colleagues who can help with specific tasks. At the macro level, this can accumulate to a big drag on organizational
productivity – valuable insights are missed, time is lost to searching, and opportunities pass by untapped.
In this post, I propose five ways AI can help you get more
value from your data.
1. Use AI to add tags and descriptions to your images so they can be searched
Most organizations have a lot of images - perhaps related to products, events, marketing assets, content for intranet news articles, or perhaps captured by a mobile app or Power Apps solution. The problem with images, of course, is that they cannot be searched easily - if you go looking for a particular image within your intranet or digital workplace, chances are you'll be opening a lot of them to see if it's what you want. Images are rarely tagged, and most often are stored in standard document libraries in Microsoft 365 which don't provide a gallery view.
A significant step forward is to use image recognition to automatically add tags and descriptions to your pictures in Microsoft 365. Now, the search engine can do a far better job of returning images - users can enter search terms and perform textual searches rather than relying on your eyeballs and lots of clicks. Certainly, the AI may not autotag your images perfectly - but the chances are that some tagging is better than none. Here are some examples I've used in previous articles to illustrate what you can expect from the Vision API in Azure Cognitive Services:
Image
|
Result
|
|
|
Image
|
Result
|
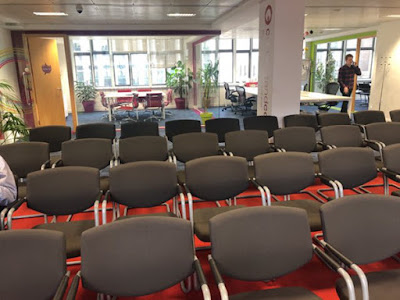
|
|
Image
|
Result
|
|
|
2. Use AI to extract entities, key phrases and sentiment from your documents
We live in a world of documents, and in the Microsoft 365 world this generally means many Teams and SharePoint sites full of documents, usually with minimal tagging or metadata. Nobody wants the friction of manually tagging every document they save, and even a well-managed DMS may only provide this in targeted areas. Auto-tagging products have existed for a while but have historically provided poor ROI due to expensive price tags and ineffective algorithms. As a result, the act of searching for information typically involves opening several documents and skimming before finding the details you're looking for.
What if we could extract key phrases from documents and known entities (organizations, products, people, concepts etc.) and highlight them next to the title so that the contents are clearer before opening? Technology has moved on, and Azure's Text Analytics API is far superior to the products of the past. In my simple implementation below I'm simply sending each document in a SharePoint library to the API and then storing the resulting key phrases and entities as metadata. I also grab the sentiment score of the document as a bonus:
A more advanced implementation might provide links to more information on entities that have been recognized in the document. The Text Analytics API has a very nice capability here - if an entity is recognized that has a page on Wikipedia (e.g. an organization, location, concept, well-known person etc.), the service will detect this and the response data for the item will include a link to the Wikipedia page:
There are lots of possibilities there of course!
3. Create searchable transcripts for old calls, meetings and webinars with speech-to-text AI
If your company uses Microsoft 365, then Stream is already capable of advanced speech-to-text processing - specifically, the capability which automatically generates a transcript of the spoken audio within a video. This is extremely powerful for recording that important demo or Teams call for others to view later of course. However, not every organization is using Stream - or perhaps there are other reasons why some existing audio or video files shouldn't be published there.
In any case, many organizations do have lots of this type of content lying around, perhaps from webinars, meetings or old Skype calls. Needless to say, all this voice content is not searchable in any way - so any valuable discussion won't be surfaced when others look for answers with the search engine. That's a huge shame because the spoken insights might be every bit as valuable as those recorded in documents.
A note on Microsoft Stream transcripts
Whilst Stream is bringing incredible capabilities around organizational video, it's worth noting that transcripts are not searched by Microsoft 365 search - only by 'Deep Search' in Stream. So if you've honed in on a particular video and want to search within it, Deep Search is effective - but if you're at step one of trying to find content on a particular topic, videos are not currently searched at the global level in this way.
Speech-only content comes with other baggage too. As just one example, it may be difficult to consume and understand for anyone whose first language is different from the speaker(s).
The Azure Speech Service allows us to perform many operations such as:
- Speech-to-text
- Text-to-speech
- Speech translation
- Intent recognition
More advanced scenarios also include call recording, full conversation transcription, real-time translation and more. In the call center world, products such as Audiocodes and Genesys have been popular and are increasingly integrated with Azure's advanced speech capabilities - indeed, Azure has dedicated real-time call center capabilities these days.
At the simpler end though, if your company does have lots of spoken content which would benefit from transcription you can do this without too much effort. I wrote some sample code against the API and tested a short recording I made with the PC microphone - I don't need to tell you what I said because the API picked it up almost verbatim:

If we're splitting hairs, really I said this as one sentence so the first full stop (period) should arguably be a comma. Certainly this was a short and simple recording, but as you can see the recognition level is extremely high - and astonishingly, the API even managed to spell O'Brien correctly!
Here's the code required to call the API, largely as described in the docs:
4. Use AI to translate documents
The case for this one is simple to understand - an organization can have a variety of reasons for translating documents, and AI-based machine translation has advanced sufficiently that it's precise enough for many use cases. Working with international suppliers or clients could be one example, or perhaps it's because search isn't effective enough in a global organization - users are searching in their language, but key content is only available in another language.
Azure allows you to translate documents individually or at scale through APIs or scripting, all in a very cost-effective way. I didn't need to build anything to tap into it, since a ready-made front-end exists in the form of the
Document Translator app in Github - once hooked up to my Azure subscription, I'm ready to go.
In this tool, if you supply a document you get a completed document back - in other words, pass in a PowerPoint deck and get a file with each slide translated in return - no need to paste anything back together. The Translator capability in Azure Cognitive Services allows you to tap into same translation engine behind Teams, Word, PowerPoint, Bing and many other Microsoft products,
but also to build your own custom models to understand language and terminology specific to your case.
My French is a bit rusty, but these look fairly good to me:
Translation of documents you already have offers lots of possibilities, with improved search being just one example. But there are many other high-value translation scenarios too, such as real-time speech translation -
something that's now possible in Teams Live Events. With Azure Cognitive Services, it's also possible to build the capability into your own apps without needing to use Teams, and you're tapping into the same back-end underneath.
5. Extract information from documents such as invoices, contracts and more
In one of the earlier examples we talked about extracting key phrases, entities and sentiment. In some cases though, the valuable content within a document is found within a certain section of the document - perhaps a table, a set of line items or grand total. Every organization in the world has loosely-structured documents such as invoices, contracts, expense receipts and order forms - but often the valuable content is deeply embedded and each document needs to be opened to get to it. With the
Forms Recogniser capability in Azure, you can use either pre-built models for common scenarios or train a custom model yourself, allowing the AI to learn your very specific document structure. This is the kind of capability that is coming in Project Cortex (essentially a version of this tightly-integrated with SharePoint document libraries), but it may be more cost-effective to plug into the Azure service yourself.
Some examples are:
- Forms - extract table data or key/value pairs by training with your forms
- Receipts and business cards - use pre-built models from Microsoft for these
- Extract known locations from a document layout - extract text or tables from specific locations in a document (including handwriting), achieved by highlighting the target areas when training the model
So if you have documents like this:
..you could extract the key data and make better use of it (e.g. store as searchable SharePoint metadata or extract into a database to move from unstructured to structured data).
Conclusion
AI is within reach now, and many of the above scenarios can be achieved without coding or complex implementation work. There needs to be a person or team somewhere who knows how to stitch together Azure AI building blocks and Microsoft 365 of course, but the complexity and cost barriers are melting away.
Beyond the scenarios I present here, lots of value can be found in use cases which combine some of the above capabilities with other actions. Some examples where you could potentially roll your own solution rather than invest in an expensive platform could be:
- Analyze call transcripts for sentiment (run a speech-to-text translation and then derive sentiment) and provide a Power BI report
- Perform image recognition from security cameras and send a push notification or post to a Microsoft Team if something specific is detected
- Auto-translate the transcript of a CEO speech or townhall event and publish on a regional intranet
The AI element of all of these scenarios and more is now easily achieved with Azure. What a time to be in technology!